|
|
SI-Cut: Structural
Inconsistency Analysis
for Image Foreground Extraction
|
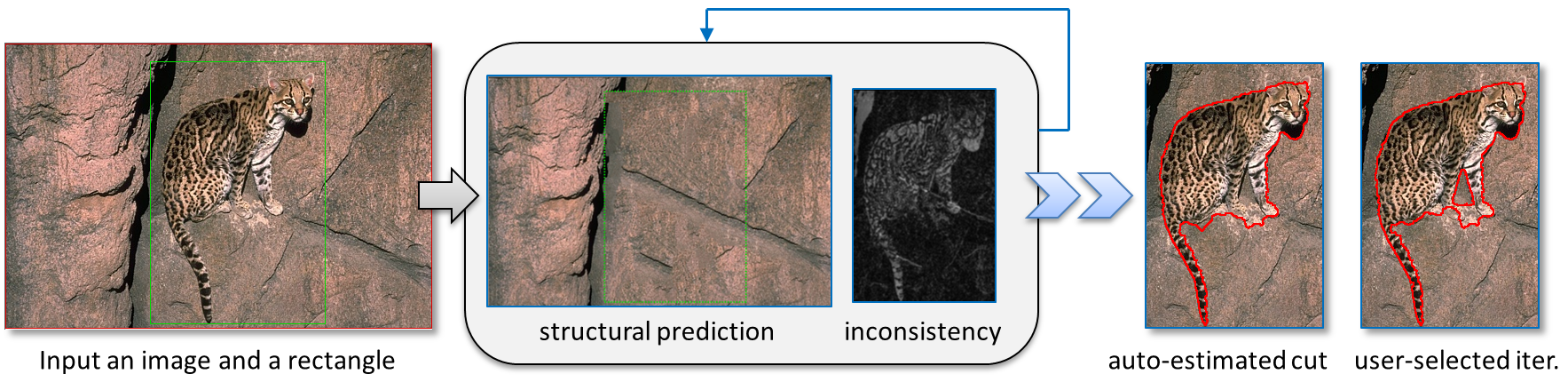
(C) CAIG Lab, NCTU |
Authors
|
I-Chen
Lin,
Yu-Chien
Lan,
Po-Wen
Cheng
Corresponding author: I-Chen Lin
|
Abstract
|
This paper presents
a novel approach for extracting foreground objects from an image.
Existing methods involve separating the foreground and background
mainly according to their color distributions and neighbor
similarities. This paper proposes using a more discriminative
strategy, structural inconsistency analysis, in which the localities
of color and texture are considered. Given an indicated rectangle,
the proposed system iteratively maximizes the consensus regions
between the original image and predicted structures from the known
background. The object contour can then be extracted according to
inconsistency in the predicted background and foreground structures.
The proposed method includes an efficient image completion technique
for structural prediction. The results of experiments showed that
the extraction accuracy of the proposed method is higher than that
of related methods for structural scenes, and is also comparable to
that of related methods for less structural situations.
|
Experiments
|
The proposed method
was compared with three related state-of-the-art methods: GrabCut
[Rother et al. 2004], the pinpoint method with a bounding box prior
[Lempitsky et al. 2009] (abbreviated as Box-prior), and the
GrabCut in one cut [Tang et al. 2013] (abbreviated as One-cut).
Several
segmentation results of the proposed and comparative methods are
shown below. Please refer to the manuscript and supplementary
files for more results and comparison.
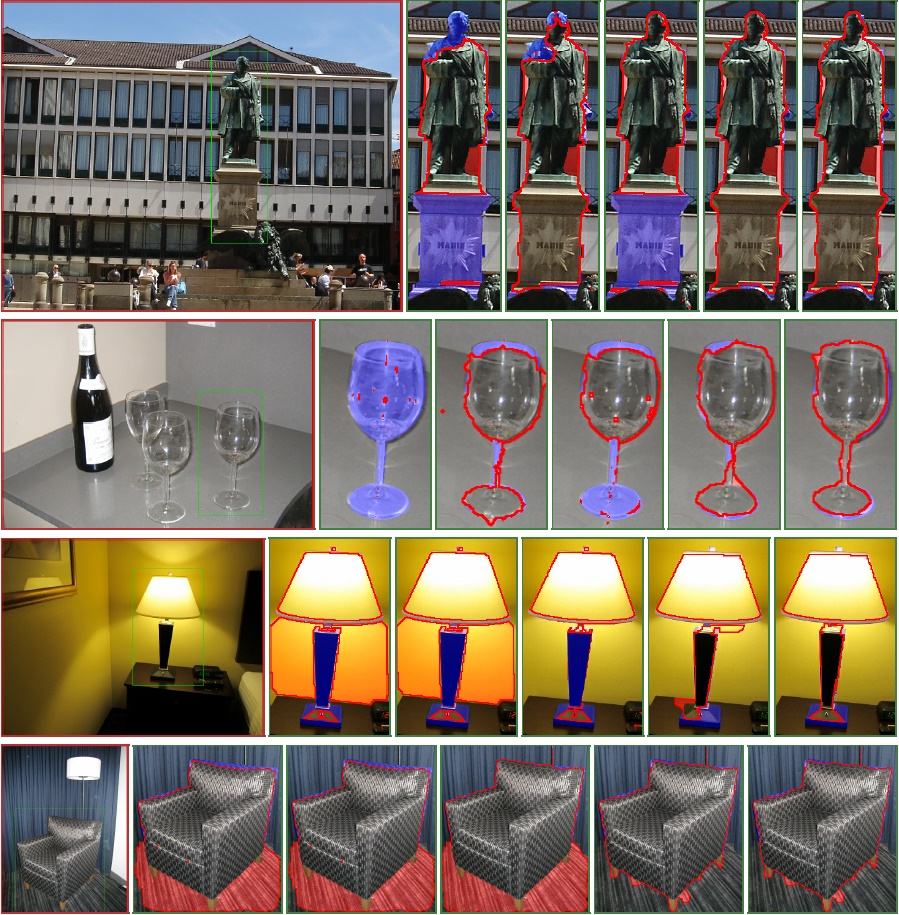
Figure.
Comparative results for the structural scene dataset. From left to
right: Input images and rectangles, GrabCut results, Box-prior
results, One-cut results (with individually optimal weights), the
proposed results (AE: with auto-estimation iterations), the proposed
results (UA: with user-assigned iterations).
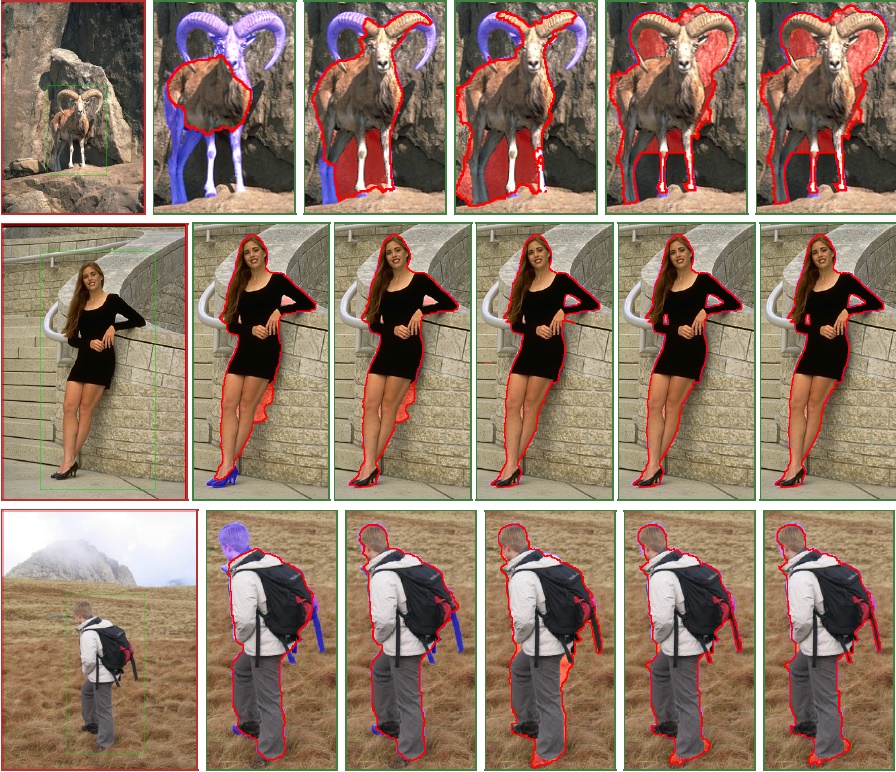
Figure.
Comparative results for the GrabCut dataset. From left to right:
Input images and rectangles, Grab- Cut results (from [Lempitsky et
al. 2009]), Box-prior results [Lempitsky et al. 2009], One-cut
results [Tang et al. 2013], the proposed (AE) results, the proposed
(UA) results.
|
Datasets used in the
experiments
|
Structural scene
dataset (SSDB40)
The 40 source images were selected from the
LabelMe
project page. The credits for the image sources go to the LabeMe
database [Russel et al. 2008]. A large portion of ground-truth masks
in LabelMe are approximated by polygonal contours. For more accurate
experiments, the ground-truth masks were refined by users and used
in this experiment.
Download:
SSDB40_pack.zip (zipped file, about 16.7MB, including images,
indicated rectangles and ground-truth masks)
SSDB40_src_mapping
(The mappings between images in SSDB40 and LabelMe are listed in
this table)
GrabCut dataset
The 50 source images and ground truth masks of GrabCut dataset are
from
GrabCut project page [Rother et al. 2004].
The indicated rectangles used in this experiment are identical to
those used in [Lempitsky et al. 2009].
(NOTE: As emphasized in [Lempitsky et al. 2009], the results
and error rates reported here are based on bounding-rectangle
inputs. They are not appropriate for comparison with results and
error rates of related methods based on the trimap, lasso or
scribble inputs.)
|
Publication
|
I-Chen Lin, Yu-Chien
Lan, Po-Wen Cheng, "SI-Cut: Structural Inconsistency Analysis for
Image Foreground Extraction," IEEE Trans. Visualization and
Computer Graphics, 21(7):860-872, July, 2015.
Paper:
preprint_version (about 19.8MB),
published version (link to the IEEE digital library)
Supplementary file:
TVCG15_sup_results (pdf, about 16.7MB)
|
BibTex
|
@article{LinTVCG15,
author = {I-Chen Lin and Yu-Chien Lan and Po-Wen Cheng},
title = {{SI-Cut}: Structural Inconsistency Analysis for Image
Foreground Extraction},
journal = {{IEEE} Transactions on Visualization and Computer
Graphics},
volume = {21},
number = {7},
pages = {860--872},
month = {July},
year = {2015},
doi = {10.1109/TVCG.2015.2396063}
}
|
Acknowledgement
|
The authors
appreciate the helpful comments from the anonymous reviewers. This
paper was partially supported by the Ministry of Science and
Technology, Taiwan under grant no. MOST 103-2221-E-009-143.
|
Go back to I-Chen Lin's
publication webpage
(English)
|
|
|
|