The 22nd Asia-Pacific Network Operations and Management Symposium
Networking Data and Intelligent Management in the Post-COVID19 Era
Tutorials
Tutorial 1
Federated Learning for Internet of Vehicles
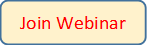
-
Date & Time: 09:00 ~ 10:30, Sept. 8, 2021.
Chair: Prof. Guey-Yun Chang (National Central University, Taiwan)
- Speaker: Dr. Celimuge Wu (Associate Professor, The University of Electro-Communications, Japan)
-
Celimuge Wu received his PhD degree from The University of Electro-Communications, Japan in 2010. He has been an associate professor of The University of Electro-Communications since 2015. His research interests include Vehicular Networks, Edge Computing, IoT, Intelligent Transport Systems, and Application of Machine Learning in Wireless Networking and Computing. He serves as an associate editor of IEEE Transactions on Network Science and Engineering, IEEE Transactions on Green Communications and Networking, IEEE Open Journal of the Computer Society, Wireless Networks, and IEICE Transactions on Communications. He also has been a guest editor of IEEE Transaction on Intelligent Transportation Systems, IEEE Transactions on Emerging Topics in Computational Intelligence, IEEE Computational Intelligence Magazine etc. He is the chair of IEEE TCGCC Special Interest Group on Green Internet of Vehicles and IEEE TCBD Special Interest Group on Big Data with Computational Intelligence. He is a recipient of 2021 IEEE Communications Society Outstanding Paper Award, and IEEE Computer Society 2019 Best Paper Award Runner-Up. He is a senior member of IEEE.
- Summary of Presentation
-
In order to support advanced vehicular Internet-of-Vehicles (IoV) applications, information exchanges among different vehicles are required to find efficient solutions for catering to different application requirements in complex and dynamic vehicular environments. Federated learning (FL), which is a type of distributed learning technology, has been attracting great interest in recent years as it performs knowledge exchange among different network entities without a violation of user privacy. This talk will focus on the applications of FL in vehicular environments in order to facilitate emerging vehicular IoV applications.
Tutorial 2
Edge Learning: Enabling Distributed Machine Learning in Cloud-Edge Environment
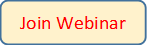
-
Date & Time: 09:00 ~ 10:30, Sept. 8, 2021.
Chair: Prof. Sangheon Pack (Korea University, Korea)
- Speaker: Dr. Peng Li (Associate Professor, The University of Aizu, Japan)
-
Peng Li received his BS degree from Huazhong University of Science and Technology, China, in 2007, the MS and PhD degrees from the University of Aizu, Japan, in 2009 and 2012, respectively. Dr. Li is currently an Associate Professor in the University of Aizu, Japan. His research interests mainly focus on cloud computing, Internet-of-Things, big data systems, as well as related wired and wireless networking problems. Dr. Li has published over 100 technical papers on prestigious journals and conferences. He won the Young Author Award of IEEE Computer Society Japan Chapter in 2014. He won the Best Paper Award of IEEE TrustCom 2016. He supervised students to win the First Prize of IEEE ComSoc Student Competition in 2016. Dr. Li is the editor of IEICE Transactions on Communications. He is a member of IEEE.
- Summary of Presentation
-
Machine learning has demonstrated great promises in various fields, e.g., finance, self-driving and healthcare. The success of deep learning stems from the availability of big training data and massive computation power. However, in many applications, training data are generated by distributed devices owned by individuals, who hesitate to share their data that expose privacy. Moreover, it becomes difficult to aggregate these data to a single computing site for centralized training due to the increasing data size. In this talk, we will present a paradigm complementary to the cloud-based methods for machine learning in the cloud-edge environment. It is proposed and developed for moving the training and inference to the edge environment to serve the delay-sensitive and privacy-sensitive applications, of which the data cannot be gathered to the cloud. While edge learning has great potential for many intelligent applications, e.g., smart cities and self-driving cars, it is quite challenging to realize it in an efficient and secure manner due to the inherent characteristics of the cloud-edge environment. We will present the opportunities, open challenges and possible solutions of edge learning in this talk.
Tutorial 3
Trajectory Design in Wireless Drone Networks: Moving from Offline Optimization to Online Learning
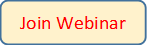
-
Date & Time: 12:40 ~ 14:10, Sept. 8, 2021.
Prof. Guey-Yun Chang (National Central University, Taiwan)
- Speaker: Dr. Yu-Jia Chen (Assistant Professor, National Central University)
-
Yu-Jia Chen received the B.S. degree and Ph.D. degree in electrical engineering from National Chiao Tung University, Taiwan, in 2010 and 2015, respectively. From 2015 to 2018, he was a postdoctoral research fellow with National Chiao Tung University, Taiwan, and he was a postdoctoral research fellow with Harvard University from 2018 to 2019. In 2019, he joined National Central University, Taiwan, where he is currently an assistant professor at the department of communication engineering. His research interests include AI-enabled communication networks, wireless sensing, and network security. Dr. Chen has published more than 30 articles in peer-reviewed international journal and conference papers. He is holding four US patents and four ROC patents. Dr. Chen has been serving as Technical Organizing Committee and Symposium Cochair for many international conferences and symposia, including Globecom, VTC, and PIMRC. He is also co-founder of the IEEE workshop SPSCS, focusing on security and privacy in smart and connected systems. Prof. Chen has experience with tutorials at academic conferences such as Globecom and VTC.
- Summary of Presentation
-
Unmanned aerial vehicles (UAVs) have recently gained wide popularity in various wireless applications due to their agility and high mobility features. In this talk, we will first review the trajectory design problem of cellular-connected UAVs. We will discuss some examples of UAV trajectory design taking into account the communication performance of the entire trajectory. Then we will look into the nature of graph theory based approaches and introduce a low-complexity method to solve the unconstrained problem by finding the shortest trajectory in an undirected weighted graph. Next, we introduce a novel imitation augmented deep reinforcement learning approach to solve the challenging connectivity- constrained problem. Finally, we provide some results from both simulated and real experiments to illustrate the effectiveness of the proposed method.
Tutorial 4
Human Behavior in the Time of COVID-19 Pandemic: Learning from Data Science
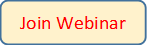
-
Date & Time: 12:40 ~ 14:10, Sept. 8, 2021.
Chair: Prof. Sangheon Pack (Korea University, Korea)
- Speaker: Dr. Jinyoung Han (Assistant Professor, Sungkyunkwan University)
-
Jinyoung Han is Assistant Professor in the Department of Applied Artificial Intelligence at Sungkyunkwan University (SKKU), where he leads the Data Science and Artificial Intelligence Lab (DSAIL). Before joining SKKU, he was Assistant Professor at Hanyang University (ERICA). He was a postdoctoral researcher at University of California, Davis, and Seoul National University. He received his Ph.D. in computer science and engineering from Seoul National University in 2013, and B.S. degree in computer science from KAIST in 2007. His research interests generally lie in data mining, network science, machine learning, and social computing.
- Summary of Presentation
-
The outbreak of COVID-19 has a significant impact on individual mental health and human behavior. As the use of social media has become a part of daily life, the posts about COVID-related stress, symptoms, and emotions could be easily observed in social media. In this talk, we will show how COVID-19 pandemic affects mental status such as emotions and suicidal ideation using social media data and NLP techniques. Also, as the COVID-19 pandemic progresses, the uncertainty of the unprecedented crisis stimulates the proliferation of fake news. This talk introduces how to detect fake news on COVID-19 using graph learning and NLP models.